Electroencephalographic Signatures as Predictors of Antidepressant Response
- Richard Nauman
- Mar 5
- 3 min read
By: Aral Cetiner
Abstract
Electroencephalography (EEG) is promising in developing personalized medicine and treating major depressive disorder (MDD). This article explores a seminal work published in Nature Biotechnology, which introduces a robust EEG-based signature capable of predicting antidepressant response specific to sertraline (Zoloft), an SSRI.1 A step forward in biomarker discovery could change clinical approaches to treating depression, strengthening tailored therapeutic strategies.
Introduction
MDD is a complex and biologically heterogeneous disorder. Traditionally, treatment responses are relatively unpredictable. For antidepressant treatment, current methodologies are primarily trial-and-error, which contributes to prolonged suffering and economic burden. The ubiquity of MDD and its treatment complications in modern society demonstrate a trend toward neuroscientific solutions. Single-dose psilocybin treatment, replicated in randomized-controlled trials (RCTs), is one example.2 The study of our focus, which is more or less a seminal work, addressed this issue by employing advanced machine learning (ML) techniques to resting-state EEG data, exposing a predictive signature for predictive responsiveness.
Methodology
A novel algorithm was developed – Sparse EEG Latent SpacE Regression (SELSER) – designed to optimize signal extraction and minimize EEG data noise. Given previous advancements, this approach was distinct in its capacity to handle the high dimensionality and inherent noisiness of EEG signals. The project harnessed data from the largest neuroimaging-coupled placebo-controlled study of antidepressant efficacy to date (n=309), utilizing cross-validation methods enhancing the robustness and applicability of findings.3

Figure 1 shows the sequential steps involved in processing pretreatment EEG data to predict treatment outcomes: spatial filtering of EEG signals from multiple electrodes and extraction of band power features, which are then used in a linear regression model to predict the treatment outcome. Convex optimization is aimed at minimizing prediction error and enhancing the accuracy and reliability of treatment outcomes based on EEG signatures.
Results
The SELSER algorithm successfully identified an EEG signature predictive of response to sertraline but not to placebo, demonstrating its specificity. This signature was validated across multiple independent datasets and demonstrated the capability to generalize across different EEG systems and clinical settings — often the problem. The predictive power of the EEG signature was thus demonstrated.
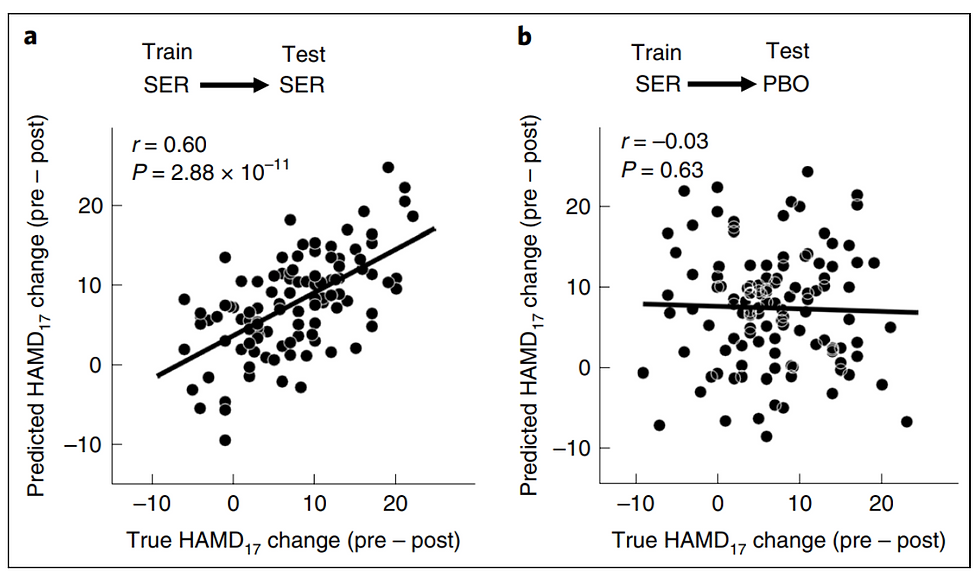
Figure 2a shows a strong predictive relationship between EEG signature and actual treatment outcomes in the setraline arm, demonstrating the effectiveness of the SELSER model. Figure 2b complements the prior, demonstrating the specificity of the model for sertraline. The predictive signature does not erroneously predict improvements in the placebo group.
Clinical Implications
By predicting antidepressant response from EEG data, clinicians can make informed decisions, hastening the process of selecting effective treatments for MDD patients. This approach aligns with the movement toward precision medicine, emphasizing customized and individually tailored healthcare.
Future Directions and Challenges
The findings of Wu and colleagues are promising; however, the scalability of such technology in clinical settings is challenging. It principally requires the integration of complex ML models and EEG analysis into routine clinical practice. Schwartzmann and colleagues mentioned similar problems, factoring in the practical application of EEG technology in clinical settings to assess the prediction of response to two distinct SSRIs.4 Future research should concentrate on expanding predictive capabilities to other antidepressant classes and investigate the underlying neurobiological mechanisms implicated by the EEG signatures.
Conclusion
The treatment of depression can be approached from several angles: EEG has potential in clinical psychiatry and may form a larger part of the personalized treatment landscape. This development also sets a precedent for how neuroscientific research can directly inform and improve clinical practices.
References
Wu W, Zhang Y, Jiang J, et al. An electroencephalographic signature predicts antidepressant response in major depression. Nat Biotechnol. 2020;38(4):439-447. doi:10.1038/s41587-019-0397-3
Haikazian S, Chen-Li DCJ, Johnson DE, et al. Psilocybin-assisted therapy for depression: A systematic review and meta-analysis. Psychiatry Res. 2023;329:115531. doi:10.1016/j.psychres.2023.115531
Trivedi MH, McGrath PJ, Fava M, et al. Establishing moderators and biosignatures of antidepressant response in clinical care (EMBARC): Rationale and design. J Psychiatr Res. 2016;78:11-23. doi:10.1016/j.jpsychires.2016.03.001
Schwartzmann B, Dhami P, Uher R, et al. Developing an Electroencephalography-Based Model for Predicting Response to Antidepressant Medication. JAMA Netw Open. 2023;6(9):e2336094. Published 2023 Sep 5. doi:10.1001/jamanetworkopen.2023.36094
コメント